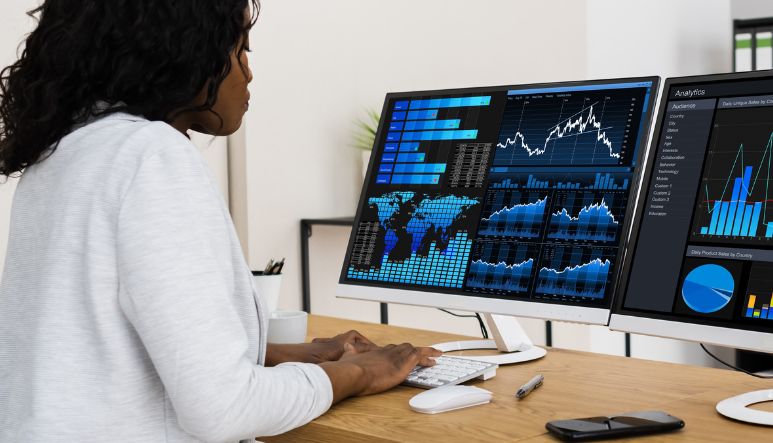
It is essential to be well informed when it comes to making credit risk judgments in supply chain finance to ensure the financial stability and growth of a company. The complexity and global nature of supply chains makes precise and educated credit risk assessment more important than ever. One effective approach is to use historical data for credit risk decision-making, which can significantly enhance accuracy, efficiency, and overall success.
The Role of Historical Data in Credit Risk Assessment
When assessing credit risk in supply chain finance, lenders must consider the possibility of borrowers failing to meet their financial obligations. This complex process involves multiple entities in the supply chain. However, historical data is a valuable resource that can inform credit decisions. By analyzing past transactions, payment histories, and credit utilization, lenders can make more informed and accurate credit risk decisions. Additionally, this data can be used to create predictive models that help lenders anticipate potential risks and opportunities. A personalized approach strengthens borrower relationships as credit terms are tailored to their financial capabilities. Analyzing historical data also allows lenders to identify risk factors and early warning signals, enabling them to proactively address emerging risks and develop strategies to mitigate potential disruptions or defaults in the supply chain.
Leveraging Historical Data for Supply Chain Finance
Collecting and combining relevant historical data from various sources within the supply chain is essential in effectively evaluating credit risk in supply chain finance. Through advanced data analytics techniques, such as machine learning and predictive modeling, lenders can analyze this data to identify patterns, correlations, and anomalies. By creating risk-scoring models based on historical data, lenders can make more informed lending decisions and classify borrowers into different risk categories. Continuously monitoring and updating historical data is crucial to adapt to changing circumstances and making timely adjustments to credit terms or risk mitigation strategies. This personalized approach can lead to better borrower relationships and prevent potential defaults or disruptions in the supply chain.
Conclusion
Looking at past financial behaviors, trends, and patterns can be a helpful tool for lenders in assessing creditworthiness in supply chain finance. By utilizing historical data through advanced data analytics techniques like machine learning and predictive modeling, lenders can take a personalized approach to make informed lending decisions. Risk-scoring models can be created based on this data, allowing lenders to categorize borrowers into different risk levels and make timely adjustments to credit terms or risk mitigation strategies. Continuously monitoring and updating historical data can lead to better borrower relationships and mitigate potential defaults or disruptions in the supply chain. Embracing this approach can be a strategic move for navigating the complexities of modern supply chain finance.
Optimizing your supply chains is crucial for enhancing operational efficiencies and propelling your business forward. ASYX specializes in co-creating tailored strategies that enable possibilities for growth. So, why wait? Contact ASYX today and pave the way for a transformative future.